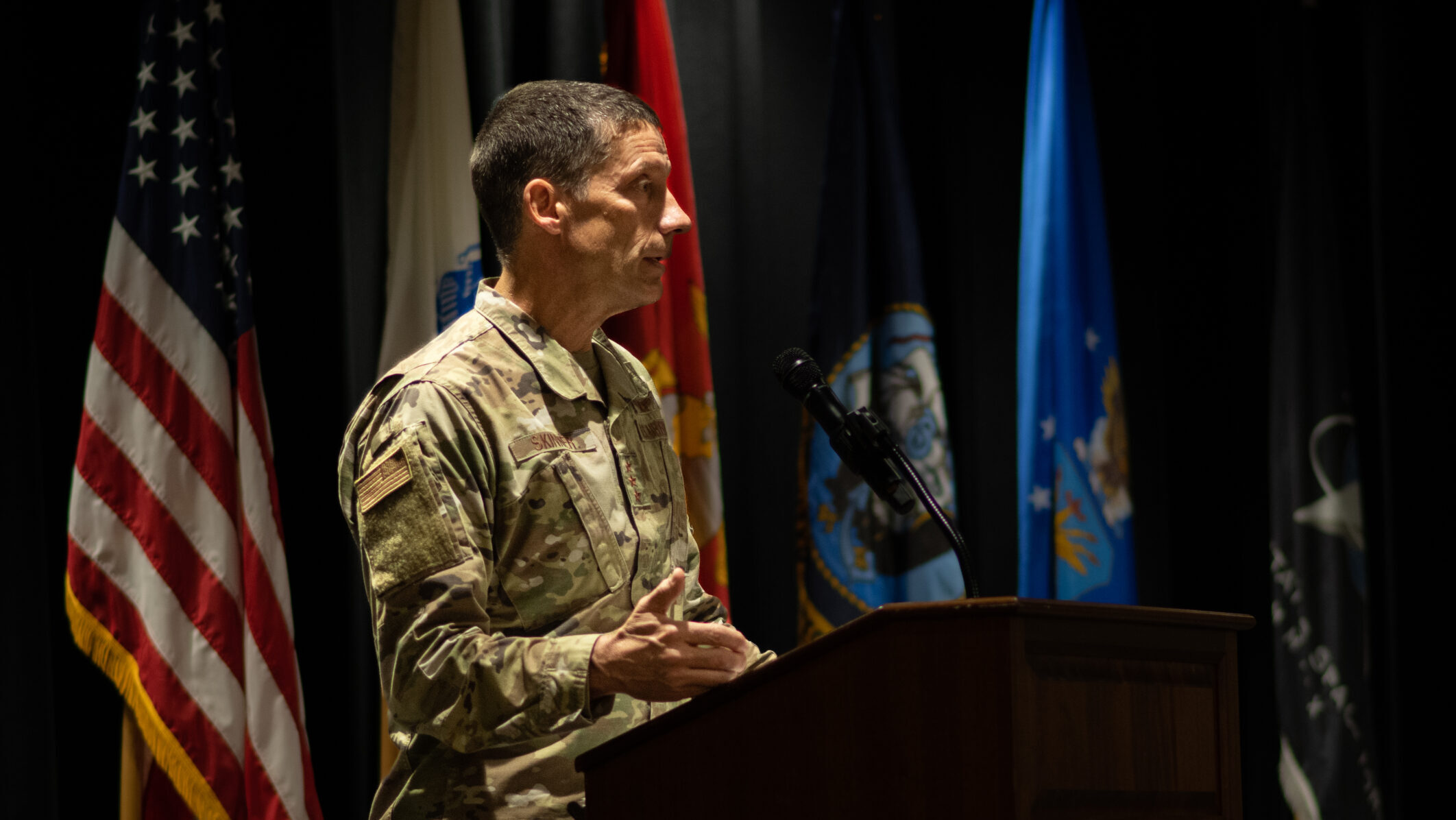
U.S. Air Force Lt. Gen. Robert Skinner, director of Defense Information System Agency and the commander of the Joint Force Headquarters, delivers remarks during the DISA Central Field Command change of command ceremony at MacDill Air Force Base, Florida, July 7, 2023. (US Air Force)
WASHINGTON — As the Pentagon struggles to catch up on generative AI, it’s wrestling with all the same challenges as the private sector — but on steroids. So while the military is eager to take advantage of civilian innovation, one senior official after another has made clear the military must implement commercial GenAI with extensive “guardrails.”
The Pentagon’s challenge is two-fold: to keep bad data from getting in and to keep DoD data from leaking out.
“How do you protect the data in the Large Language Models?” asked Lt. Gen. Robert Skinner, director of the Defense Information Systems Agency (DISA), at an AFCEA conference in March. “We’re actually working with DARPA right now … to figure out, how can DoD have one that is protected, [that] takes advantage of Large Language Models externally, but doesn’t have our data filter out.”
Skinner’s concern? Many commercially available LLMs, ravenous for ever-larger amounts of data to train their next-generation algorithms, suck up their users’ inputs and add them to that training data. To paraphrase Nietzche, when you use a chatbot, the chatbot may also be using you: It’s collecting information on its users at the same time they’re getting information from it.
“You’re not simply taking data one-way. There’s a two-way connection,” said Winston Beauchamp, Deputy CIO for the Department of the Air Force, at the same event. “You’re essentially contributing to the corpus [of data] that’s being used to train the system for everybody else.”
That’s particularly problematic for the Pentagon, because a high-end user’s query may be much more than “recommend good Chinese restaurants in Cleveland” or “write a middle-school essay about Love’s Labor’s Lost.” It may include paragraphs of context and detailed scenarios for the LLM to analyze.
So while LLMs can go wrong by “hallucinating” false answers, they can also go horribly right by learning so much correct information that they become a security risk.
“One of the first things we did with an LLM when we started playing around with it…we were quickly able to get it to hallucinate, by just feeding it some bad data sets,” said Stephen Wallace, Lt. Gen. Skinner’s CTO at DISA, speaking at a Potomac Officers’ Club conference the following day.
RELATED: ‘Poisoned’ data could wreck AIs in wartime, warns Army software acquisition chief
“The flipside is the capture of that model,” Wallace went on. “[If] I’m going to take this model and I’m going to feed it a bunch of my most critical data, then how I am going to make sure that model doesn’t walk right out the door?”
That means it’s not enough for DoD to protect its data: It also must protect the algorithms trained on that data, because they could be reverse-engineered to divulge it.
The first, most basic precaution is to avoid using publicly accessible models, which is harder than it may sound. Much like the Wizard of Oz with its infamous “man behind the curtain,” many companies offering “AI” products have actually just built an attractive custom interface that, hidden from the user, delegates all the actual work to one of the big commercial LLMs.
“We’ve many times seen front ends hosted in govcloud environments with them querying LLMs that sit out in the commercial space,” Wallace said. “That is something that we don’t allow….We don’t want the warm and fuzzy [reassurance] of ‘oh, it’s hosted in govcloud’ only to find the data is streaming out the back.”
So the Pentagon’s preferred solution is to have the model running on an isolated, DoD-only network. Isolation reduces the risk of it leaking data to the outside, making it (relatively) safe to feed the LLM sensitive information to fine-tune its responses. Isolation also helps the hallucination problem, since DoD can put the algorithms on strict data diet: Instead of letting the LLM generate answers based on whatever it gleaned from the open internet, it can be fed carefully curated and vetted data that DoD actually trusts.
One leading approach to data diets is called Retrieval-Augmented Generation. In essence, RAG connects a Large Language Model to a specific database and makes it “look up” its answers in that database, rather than letting it rely on whatever it learned from the open internet. (RAG can even require the LLM to generate verifiable citations with links to sources). As one IBM blog put it, an unaided LLM is like a student relying on their memory to pass an exam, while an LLM with RAG gets to consult the textbook.
If an LLM is left to its own devices with insufficient data, “it will try very hard to make something up that sounds reasonable and compelling,” said John Beieler, assistant director for Science and Technology at the Office of the Director of National Intelligence. “[We’re] focusing on things like RAG so we’re actually grounding it in reporting, in intelligence data… … things that exist.”
Techniques like RAG have another major benefit for the Defense Department: It means DoD doesn’t need to build its own Large Language Models from scratch in a secure, isolated environment. Instead, it can buy the latest and greatest models from the commercial world, create a slimmed down, self-contained copy that can run on a secure networks — a process called containerization — and then customize that containerized version by feeding it DoD-specific data.
“Do we really need a DoD-scale foundational model?” asked Navy Capt. M. Xavier Lugo, chief of the Pentagon’s generative AI study group, Task Force Lima, at a Pentagon CDAO event in February. “I don’t know yet, [but] they’re fricking expensive to build, and so, am I really going to justify spending that money on something I’m not 100% sure we need? I don’t think so.”
“Now, that said, there might come a day when we have to,” Lugo caveated. “We’re just not ready.”
“I don’t know that it makes sense for the IC to train its own foundation model from scratch,” echoed ODNI’s Beieler. “[Let’s] use the foundation models trained on the broad corpus that is the internet, [with] fine-tuning on areas we have unique datasets.”